ImageNet Roulette is a web-based app that allows users to upload an image of themselves and then see what results come up when the app runs the image through its machine learning algorithm. The app was created by artist Trevor Paglen and programmer-artist Dan Shiffman.
The goal of the app is to show how machine learning algorithms can be biased, and to start a conversation about why that bias exists.
Exclusive Bonus on Real Money Roulette - Reliable US Casinos:
-
500% + 150 FS 1st Deposit
-
-
When you first visit ImageNet Roulette, you’re presented with a blank Canvas and the instruction to “Upload an image.” Once you select and upload an image, the app runs it through its machine learning algorithm.
The results are displayed on the screen, along with the percentage confidence that the algorithm has in its results.
PRO TIP:ImageNet Roulette is an online tool that uses machine learning to categorize and label images. It takes a user-uploaded image and attempts to classify it into one of the 1000 categories in ImageNet, a large database of images used for computer vision research. By using this tool, users can gain insight into the way machines interpret images and the implications of this technology.
The app pulls its data from ImageNet, a database of images that’s often used to train machine learning algorithms. ImageNet was created by Stanford University in 2009 and contains more than 14 million images.
It’s organized into more than 20,000 different categories, each of which has thousands of images.
The app doesn’t just display the results of its machine learning algorithm; it also shows how those results compare to what humans would classify the same image as. For example, if the app thinks an image is of a “monkey,” it will also show how confident humans are that the image is of a monkey.
In many cases, the app’s results are very close to what humans would classify. But in some cases, there are significant differences.
The goal of ImageNet Roulette is to start a conversation about why these biases exist in machine learning algorithms. The developers hope that by showing how these algorithms can be biased, we can start to understand why that bias exists and what we can do about it.
9 Related Question Answers Found
Wiki Roulette is a game played by two or more people using a computer. The players take turns making up fake Wikipedia articles, and the other players have to try to guess which one is real. The game is similar to the card game known as bullshit, except that instead of trying to fool your opponents with false information, you’re trying to trick them with an article that looks like it could be real, but is actually made up.
Exclusive Bonus on Real Money Roulette - Reliable US Casinos:
500% + 150 FS 1st Deposit
Ducky Luck Review
Platinum Reels Casino Review
Diamond Reels Casino Review
Wiki Roulette can be played with any number of players, but it’s best with four or more.
Pico roulette is a casino game that is played on a small, circular table. The game is similar to regular roulette, but the betting process and payoff odds are different. In pico roulette, the dealer spins a ball around the outside of the table.
In short, comp roulette is a system where you can earn points or rewards for playing casino games. The more you play, the more points you earn, which can be redeemed for various prizes or used to get discounts on future play. While the concept is simple enough, there are a few things to keep in mind if you want to make the most of your comps.
Exclusive Bonus on Real Money Roulette - Reliable US Casinos:
500% + 150 FS 1st Deposit
Ducky Luck Review
Platinum Reels Casino Review
Diamond Reels Casino Review
First, it’s important to remember that not all casinos offer comp roulette.
La Partage Roulette is a type of roulette that is commonly found in online casinos. It is a variation of the French game, and it shares many similarities with the European version of the game. The main difference between the two games is the La Partage rule, which gives players the opportunity to recover half of their bet if the ball lands on zero.
In CSGO Roulette, players bet on which color they think the next round’s winning spin will land on. There are usually only three colors to choose from – red, black, and green – and if a player guessed correctly, they will win their bet multiplied by the odds of that color. For example, if the black color pays 2x and a player bet $100 on it, they would win $200 if it won.
Exclusive Bonus on Real Money Roulette - Reliable US Casinos:
500% + 150 FS 1st Deposit
Ducky Luck Review
Platinum Reels Casino Review
Diamond Reels Casino Review
PRO TIP:CSGO Roulette is a game based on chance where players bet on a specific color and number combination in order to win.
Rolex is a Swiss watch company that was founded in 1905 by Hans Wilsdorf and Alfred Davis. The company is known for its luxury watches, which are often used as status symbols. Rolex is also known for its innovation, as it was the first watch company to mass-produce the wristwatch and the first to use the datejust feature on a watch.
RNG roulette is a form of online gambling that uses Random Number Generation (RNG) technology to determine the outcome of each spin. RNG roulette is different from traditional online roulette in that the results are not determined by a physical wheel and ball, but rather by a computer algorithm. This makes RNG roulette a much more fair and random game, as there is no way for the casino to rig the results in their favor.
Exclusive Bonus on Real Money Roulette - Reliable US Casinos:
500% + 150 FS 1st Deposit
Ducky Luck Review
Platinum Reels Casino Review
Diamond Reels Casino Review
PRO TIP:RNG Roulette is a casino game based on random number generation.
Roulette is a casino game named after the French word meaning little wheel. In the game, players may choose to place bets on either a single number, various groupings of numbers, the colors red or black, whether the number is odd or even, or if the numbers are high (19–36) or low (1–18). The croupier then spins a wheel in one direction and a ball in the other direction around a tilted circular track running around the circumference of the wheel.
Place roulette is a game that can be played online or offline. It is a game of chance where players bet on where a ball will land on a spinning wheel. The wheel has numbered pockets, and if the ball lands in the pocket that the player has bet on, they win.
Exclusive Bonus on Real Money Roulette - Reliable US Casinos:
500% + 150 FS 1st Deposit
Ducky Luck Review
Platinum Reels Casino Review
Diamond Reels Casino Review
There are many different variations of place roulette, but the most common is American roulette.
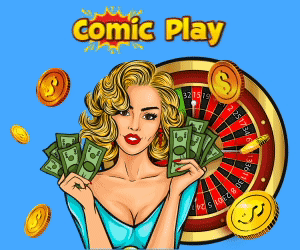